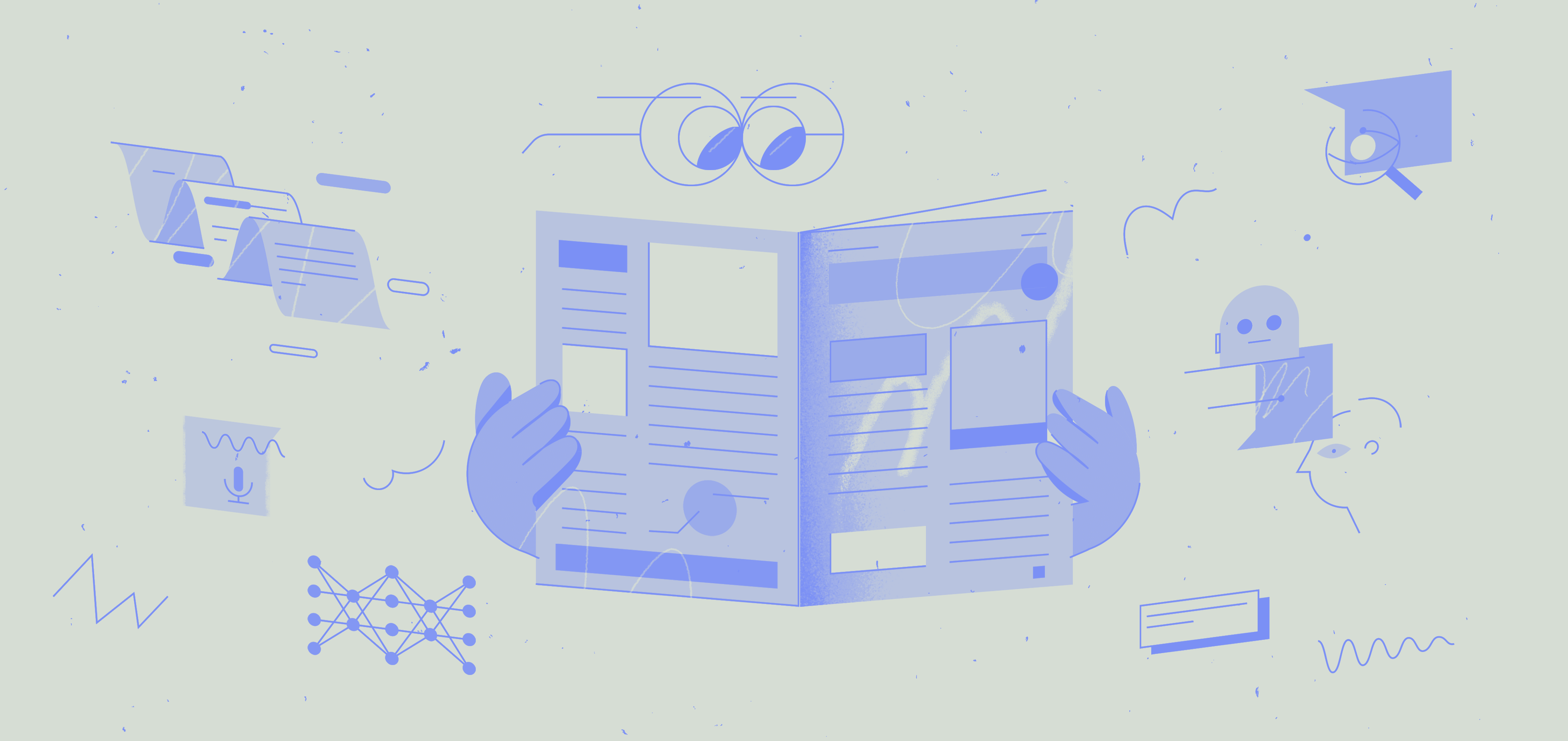
It seems April was the time for the EU to do a deeper dig into the complicated and challenging landscape of Artificial Intelligence (AI) regulations. In a world that rapidly loses trust in each new technology, it is an interesting move – and you can read more on this subject in our news digest.
While the regulatory issues are news of significance, April has also brought interesting insights into the reality of AI use in companies.
EU Shows Draft Of AI Regulations, Up To 6% Turnover Fines For Violation
The European Union has shown its strict attitude towards tech giants and its dedication toward regulating technology-related fields. With the increasing impact of AI-related technologies and solutions on people’s daily lives, the need to deliver a solid and reliable legal framework to operate has been on the rise.
In the proposition, the EU seeks to ban the use of AI-based solutions in surveillance. In doing so, the new regulation draft defines tracking individuals to rank and score their behavior. Doing so would result in severe fines of up to 6% of global turnover.
“The use of artificial intelligence for the purposes of indiscriminate surveillance of natural persons should be prohibited when applied in a generalized manner to all persons without differentiation,” the document said.
The motivation behind this rule is to avoid situations when using AI-based solutions would support existing fallacies and biases, as can be seen in the infamous Amazon’s hiring AI that was discriminating against female engineers. The same situation may occur in bank scoring or in other situations when an individual comes from an underprivileged background.
The only exceptions are made for the sake of public security reasons.
More information about the regulation can be found in Reuter’s information and in the proposal itself.
Only 6% Of Companies Have Adopted AI, 13% Are Delivering On Their AI Strategy
Over 95% of C-level executives surveyed by Juniper Networks claim that adopting AI-based solutions would greatly benefit their business. But in that same group, only 6% claim to have adopted such solutions business-wide.
What’s more, 88% of respondents claim they will use AI-based solutions, “as much as possible.” But despite this strong backing from top executives, only 22% of organizations use AI to automate or aid in the decision-making processes of their employees.
The report names three main challenges to consider:
- The lack of AI-ready technology stacks
- An insufficient supply of AI tech talents
- The lack of AI government standards
But these are not the only challenges related to building business-ready AI solutions.
The Data Problem
Another challenge in using AI on a daily basis is in the need to organize and manage owned data in a conscious and actionable manner. And while the term “big data” has been around for a long time, the majority of companies still lack the basic ability to deal with their data.
According to the MIT technology review, only 13% of surveyed companies deliver on their data strategies. The lack of standards and data architecture is named as the main challenge among multiple obstacles in building a data-oriented business culture.
Considering that, building a Big Data-powered enterprise is a step that is still to be made by many companies, and adopting AI-based technologies company-wide is the next step to be taken on that path.
Facebook Works On An AI To Predict Drug Combinations
The vast complexity of human biology delivers a significant challenge in delivering new drugs or in combining existing ones to deliver new treatments. A good example of usage AI in healthcare comes from cancer treatments where using a combination of existing drugs, instead of using only one, significantly increases the effectiveness of the treatments. Also, testing 100 new combinations of drugs takes a lot of lab time executed by highly skilled scientists. And thus, it is also costly.
Facebook researchers, along with Helmholtz Zentrum München, have delivered an open-sourced AI model used to predict favorable drug combinations (sometimes called “drug cocktails”) to increase the effectiveness of drug treatments.
The model was trained in memorizing and understanding the effects of billions of possible drug combinations on various cell types and can be used to quickly test multiple hypotheses in order to find the most effective way of treating a particular patient.
Edelman: Trust In Tech At A 21-Year Low
The Edelman trust barometer is a widely recognized, cyclical research program that examines public trust toward brands or concepts. This year’s edition focuses on technology and the fact that tech-related companies are losing the societal allure they used to have.
The year 2020 brought an initial surge in trust in tech companies due to the lockdown and the fact that the majority of the world became dependent on digital tools. With meetings and whole companies going digital, this dependence has become even stronger.
Yet this trust has eroded quickly. In the US, tech has slipped to the ninth most trusted sector, a significant slide since the year before when it held the top position. This fear is fueled by pandemic-induced job losses that have been further reinforced by the fear of AI replacing workers.
This situation creates an interesting context for the EU’s legal initiatives to regulate AI and machine learning technologies. Creating a trusted and reliable framework for the technology delivers wider social acceptance.
Earth Species Project: Working On Decoding The First Nonhuman Language
Google has already developed a system that can translate from an “image map” to a language map. Given enough training data, AI algorithms can extract semantic meaning from a range of non-linguistic inputs. Thus, even without prior knowledge, the algorithm would be able to decipher morse code, sooner or later.
A significant step forward is the Earth Species Project, an organization founded in San Francisco that is developing AI approaches such as this aimed towards communication with animals. Britt Selvitelle, the founding member, thinks that decoding the first nonhuman language is a goal that can be reached within five to ten years.
The lack of correspondence between human and animal words may not be an issue for AI because, in theory, a machine-learning system is particularly well suited to the problem of translating animalese. As long as there is some aspect of animal communication that represents something that human language can also express, there is a good chance that the AI algorithm will be able to spot it. After all, the machine is not a human, in the end.
The attempt to find such an overlap is a collaboration project of Earth Species called Whale-X. Its purpose is to collect and analyze call communication among a pod of whales during a full season, in order to identify the parts in common between human and whale languages.